Conditional versus marginal estimators of within-pair effects of exposure on outcomes in individually-matched case-control studies and twin cohorts

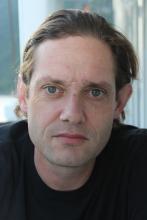
Data from individually-matched case-control studies, cohorts of twins and other paired designs provide a powerful resource that can be used estimate the magnitude of exposure-outcome associations free from confounding by shared factors. For binary outcomes, these data are typically analysed using conditional logistic regression (CLR), which uses only data from outcome-discordant pairs and, for binary exposures, also requires that pairs are exposure-discordant. An alternative is to fit an ordinary (unconditional or marginal) logistic regression (OLR) model that includes terms for between-pair and within-pair regression effects. Estimates of the within-pair regression coefficient from the OLR are potentially more efficient that the corresponding estimate from CLR since all exposure-discordant pairs contribute to the within-pair estimate regardless of whether they are outcome-discordant or not. We compare closed-form expressions and variances for estimators of the within-pair effect based on CLR and three OLR models where the pair-mean exposure is (i) assumed not to be associated with the outcome; (ii) assumed to be linearly associated with the log-odds of the outcome; (iii) included as a categorical variable to allow for an unstructured between-pair relationship. I’ll show that the within-pair estimators from these regression models are special cases of a formula based on weighted counts from a 2 × 2 exposure-outcome contingency table for exposure-discordant pairs, generalising the results of Sjölander et al. (Stat. Sci., 2012).
In this presentation I’ll review the 50-year history of modelling between- and within-cluster regression effects, from econometrics through sample surveys to biostatistics, show that in many cases we can prove that conditional and marginal estimates of within-pair regression effects are not the same, and suggest when the marginal estimator is likely to offer increased efficiency at the expense of additional assumptions about the functional form of the between-pair regression effect. I’ll foreshadow future work extending the theory to accommodate larger sib-ships, two time-points or “pairs of pairs”, and longitudinal studies.