Special Issue of Biometrical Journal for ISCB ASC 2018
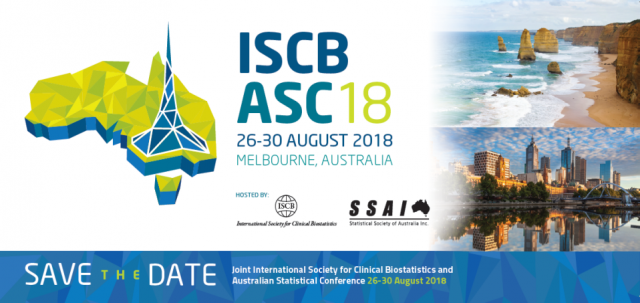
Editorial from ViCBiostat Executive, and new research from PhD candidates Marnie Downes and Mr Monsurul Hoq.
Editorial: A potpourri of biostatistical research
The motivation and themes for a Special Issue of arose from a joint conference that combined the annual meeting of the International Society for Clinical Biostatistics (ISCB) and the biennial Australian Statistical Conference 2018, held in Melbourne August 26–30, 2018.
This was an important conference for extending the international reach of the ISCB, being the first time in its 39 years that the conference had been held in the southern hemisphere, and only the third time it had been held outside of Europe. It was also an important event for Australia, highlighting the recent growth of biostatistics Down Under, albeit in the face of relatively underdeveloped foundations and institutional support.
Read the full editorial here.
Multilevel regression and poststratification as a modeling approach for estimating population quantities in large population health studies: A simulation study
Abstract
There are now a growing number of applications of multilevel regression and poststratification (MRP) in population health and epidemiological studies. MRP uses multilevel regression to model individual survey responses as a function of demographic and geographic covariates. Estimated mean outcome values for each demographic–geographic respondent subtype are then weighted by the proportions of each subtype in the population to produce an overall population‐level estimate. We recently reported an extensive case study of a large nationwide survey and found that MRP performed favorably compared to conventional survey sampling weights for the estimation of population descriptive quantities in a highly selected sample. In this study, we aimed to evaluate, by way of a simulation experiment, both the accuracy and precision of MRP versus survey sampling weights in the context of large population health studies. While much of the research into MRP has been focused on U.S. political and social science, we considered an alternative population structure of smaller size and with notably fewer geographic subsets. We explored the impact on MRP performance of sample size, model misspecification, interactions, and the addition of a geographic‐level covariate. MRP was found to achieve generally superior performance in both accuracy and precision at both the national and state levels. Results were generally robust to model misspecification, and MRP performance was further improved by the inclusion of a geographic‐level covariate. These findings offer further evidence that MRP provides a promising analytic approach for addressing participation bias in the estimation of population descriptive quantities from large‐scale health surveys and cohort studies.
Read the full article here.
Multiple imputation methods for handling incomplete longitudinal and clustered data where the target analysis is a linear mixed effects model
Abstract
Multiple imputation (MI) is increasingly popular for handling multivariate missing data. Two general approaches are available in standard computer packages: MI based on the posterior distribution of incomplete variables under a multivariate (joint) model, and fully conditional specification (FCS), which imputes missing values using univariate conditional distributions for each incomplete variable given all the others, cycling iteratively through the univariate imputation models. In the context of longitudinal or clustered data, it is not clear whether these approaches result in consistent estimates of regression coefficient and variance component parameters when the analysis model of interest is a linear mixed effects model (LMM) that includes both random intercepts and slopes with either covariates or both covariates and outcome contain missing information. In the current paper, we compared the performance of seven different MI methods for handling missing values in longitudinal and clustered data in the context of fitting LMMs with both random intercepts and slopes. We study the theoretical compatibility between specific imputation models fitted under each of these approaches and the LMM, and also conduct simulation studies in both the longitudinal and clustered data settings. Simulations were motivated by analyses of the association between body mass index (BMI) and quality of life (QoL) in the Longitudinal Study of Australian Children (LSAC). Our findings showed that the relative performance of MI methods vary according to whether the incomplete covariate has fixed or random effects and whether there is missingnesss in the outcome variable. We showed that compatible imputation and analysis models resulted in consistent estimation of both regression parameters and variance components via simulation. We illustrate our findings with the analysis of LSAC data.
Read the full article here