On parameter orthogonality and proper modelling of dispersion in Poisson-inverse Gaussian regression

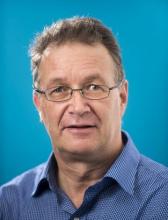
In clinical trials one traditionally models the effect of treatment on the mean response. The underlying assumption is that treatment affects the response distribution through a mean location shift on a suitable scale, with other aspects of the distribution (shape/dispersion/variance) remaining the same. This work is motivated by a trial in Parkinson’s disease patients where the primary endpoint is the number of falls during 10 weeks. Inspection of the data reveals that the Poisson-inverse Gaussian (PIG) distribution is appropriate, and that the experimental treatment reduces not only the mean, but also the variability, substantially. The conventional analysis assumes a treatment effect on the mean, either adjusted or unadjusted for covariates, and a constant dispersion parameter. On our data, this analysis yields a non-significant treatment effect. However, if we model a treatment effect on both the mean and dispersion parameters, both effects are highly significant. A simulation study shows that if a treatment effect exists on the dispersion parameter and is ignored in the modelling, estimation of the treatment effect on the mean can be severely biased. We show further that if we use an orthogonal parametrisation of the PIG distribution, estimates of the mean model are robust to misspecification of the dispersion model. We will also discuss inferential aspects that are more difficult than anticipated in this setting. These findings have implications in the planning of statistical analyses for count data in clinical trials.
Joint work with Gillian Heller (Macquarie University) and Dominique Couturier (Cambridge University)