Registration now open for ViCBiostat Summer School 2023
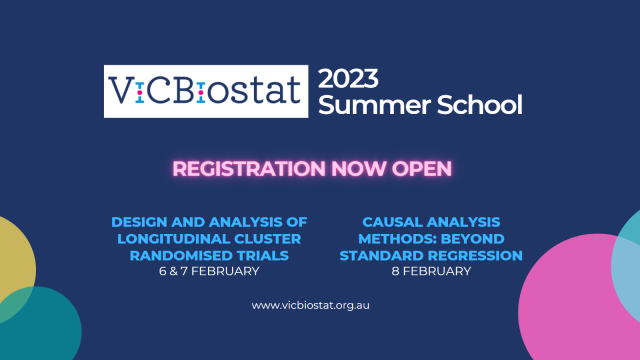
We are pleased to be returning to in-person events next year (with an option to join remotely via Zoom). Both workshops will be held at the Ground Floor Conference Rooms, Monash University SPHPM, 553 St Kilda Road Melbourne.
Please note the following new dates for our course offerings in 2023. This is an update to the dates previously advertised on the 16th of November.
We hope you will be able to join us!
Design and analysis of longitudinal cluster randomised trials
Led by Jessica Kasza
Monday 6th and Tuesday 7th February, 10am-4pm
*updated from the previously advertised dates of Thursday 9th and Friday 10th February.
Cluster randomised trials are often conducted when randomising individuals to treatments isn’t possible. This can occur when the treatment is delivered at the cluster level, but we still measure outcomes on individuals. For example, if we want to assess the impact of a new hospital-wide cleaning program on the number of patients treated at that hospital who acquire infections, we can’t randomise the individual patients to cleaning programs, and instead must randomise the entire hospital. Longitudinal cluster randomised trials extend cluster randomised trials over time, and clusters may switch between the control and intervention conditions. The stepped wedge design and cluster randomised crossover design are key examples of these trials.
In this workshop, you will learn how to design and analyse longitudinal cluster randomised trials, including the stepped wedge and cluster randomised crossover design. You will learn about the latest developments in these trials, including complex within-cluster correlation structures, “incomplete” stepped wedge designs such as the staircase design, and what can go wrong when these trials are not designed and analysed appropriately. Computer practicals will give you hands-on experience in designing and analysing longitudinal cluster randomised trials, using Stata or R.
The target audience is statisticians and researchers with some statistical background. Knowledge of mixed effects models will be assumed.
Causal analysis methods: beyond standard regression
Led by Margarita Moreno Betancur
Wednesday 8th February, 9am-5pm AEDT
In this era of “data science” it is vitally important to clearly articulate the questions that we ask of data, understand the challenges inherent in answering different types of questions, and ensure that our analysis methods are suitably aligned. An overwhelming number of clinical and public health research studies ask causal (“what if…”) questions, about the effects of treatments, policies, behaviours and other exposures on health outcomes. Answers to these questions are key to guiding decision making in health policy and practice when the goal is to improve patient outcomes and population health. Causal inference requires carefully structured reasoning to guide appropriate statistical analysis.
This workshop provides an overview of key concepts relating to the design of causal analyses and reviews the standard regression method for estimating causal effects, before moving on to the main focus which is on two alternative estimation methods that are less prone to bias: g-computation and inverse probability (or “propensity score”) weighting (IPW). Lectures and tutorials will help ground understanding of these methods, emphasising underlying intuition and assumptions, whilst a hands-on computer practical (in R and Stata) will cover their implementation in practice. All lectures and tutorials include illustrations from real-world observational epidemiological studies. Electronic copies of presentation materials will be made available online.
Prerequisites: The target audience is statisticians and researchers with some statistical background, including some knowledge of regression methods. It is desirable that participants are familiar with the distinction between the three types of research question (description/prediction/causal inference) and the target trial framework for defining causal effects and designing causal analyses (such as covered in the course "Observational studies: Modern concepts & analytic methods" delivered by the Clinical Epidemiology and Biostatistics Unit (CEBU) at the Melbourne Children’s campus).
For the computer practical, students must also have a sound working familiarity with Stata or R and have the corresponding software installed on their computer or laptop.