Why we need hazards (and why their ratios may display causal intention-to-treat effects)
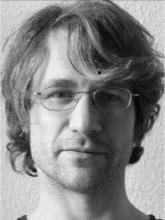
Survival or time-to-event analysis is a key discipline in biostatistics, e.g., put to prominent use in trials on treatment of and vaccination against COVID-19. A defining characteristic is that participants have varying follow-up times and outcome status is not known for all individuals. This phenomenon is known as censoring. If time-to-event and time-to-censoring are entirely unrelated, it is rather easy to see that hazards remain identifiable from censored data, and hazard estimators may subsequently be transformed to recover probability statements. However, COVID-19 treatment (because of competing risks) and vaccination trials (because of event-driven censoring) are just two of the many examples where event and censoring times are related.
Luckily, the modern counting process approach to survival analysis finds that hazards remain identifiable under rather general "independent censoring" mechanisms, including those encountered in COVID-19 trials. Given the theoretical and practical relevance of censoring, it is rather disturbing to find - as I will demonstrate - that there is a Babylonian confusion on "independent censoring" in the textbook literature. Unfortunately, censoring processes as in COVID-19 trials are two examples where the textbook literature often goes haywire. It is a small step from this mess to misinterpretations of both hazards and censoring. On the other hand, there currently is a very active debate about the use of hazards spearheaded by causal reasoning. In a nutshell, the worry is that hazards are conditional quantities which renders causal conclusions impossible ("collider bias").
I will argue that causal reasoning somewhat overfocusses on interventional "do(no censoring)" effects (which is not what identifiability of hazards is about) and that the collider bias issue disappears from a functional point of view, but that hazards remain rather subtle quantities. Time permitting, I will illustrate matters with a causal g-computation-/Aalen-Johansen-type analysis of clinical hold in a randomized clinical trial.
Jan Beyersmann is based at Ulm University Institute of Statistics where he has been a Professor of Biostatistics since 2013. His primary research focuses on survival and event history analysis, as well as statistical methodology for clinical and epidemiological studies. He has authored over 170 research papers and books Competing Risks and Multistate Models with R and Classical regression models for competing risks. Handbook of Survival Analysis 2014.
He is a Former Associate Editor of Annals of Applied Statistics, Biometrical Journal, Biometrics, Biostatistics and Statistics in Medicine; and member of the Advisory Board of the German Region of the International Biometric Society (2013-2017). Prior to his role at Ulm University, Jan held positions at the University of Freiburg, Freiburg University Hospital and Beiersdorf Research Centre.
This presentation will be streamed via Zoom and will be recorded.
Join the meeting here
Or to https://monash.zoom.us/join and enter
Meeting ID: 837 7780 6589
Passcode: 261680