The Rise of the Machines: multiple imputation and machine learning
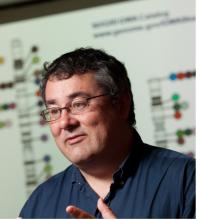
Multiple imputation is an important technique for reducing the bias caused by missing data, but it only works if it is used. Automated predictive modelling techniques from machine learning are a promising way to support semi-automated multiple imputation of large data sets. I will talk about two areas of research. First, using ensembles of trees for prediction gives imputation approaches that are competitive with standard methods in quality and scale better with increasing data size. Second, neural networks, which can make up convincing images and text, have the potential to create high-quality imputations. They are not yet ready for use by non-specialists. One of the difficulties in using machine learning for multiple imputation is that the goals of traditional prediction imply different bias/variance/parsimony tradeoffs from the goals of imputation. This is joint work with Yongshi Deng and Keiran Shao.
Thomas Lumley is Professor of Biostatistics in the Department of Statistics at the University of Auckland. He was previously Professor in the Department of Biostatistics at the University of Washington, Seattle, but is originally from Melbourne. Thomas has broad research interests in theoretical and applied biostatistics, and maintains the 'survey' package for R. He is interested in public understanding of statistics, and writes for the blog StatsChat (statschat.org.nz) and on more technical topics at notstatschat.rbind.io
Please join us for morning tea after the seminar, from 10:30am - 11:30am. No RSVP is required.
This presentation will also be accessible over Zoom and will be recorded. Please click this URL to join.
https://monash.zoom.us/j/87470193138?pwd=bmpGN1ltZlhuQzV0c1NBY0IxeklOQT09
Or, go to https://monash.zoom.us/join and enter
meeting ID: 874 7019 3138
passcode: 525580