Causal Mediation, Separable Effects, and Time
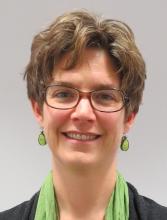
Abstract: In this presentation I will discuss 'separable effects' as an alternative approach to causal mediation analyses with view to time-dependent settings. The basic idea was introduced by Robins & Richardson (2011) and refers to a way of elaborating our causal model in order to better motivate a mediational research question. Specifically, separable effects are concerned with situations where the treatment (or exposure) can be decomposed into two or more components that could (more or less hypothetically) be intervened upon separately and thus separately 'activate' different causal pathways. It is appealing to formulate mediational research questions in this way as it sidesteps 'cross-world' notions and assumptions involved in the analysis of natural (in)direct effects based on nested counterfactuals (Andrews & Didelez, 2021).
Crucially, separable effects turn out to be especially useful in time-dependent setting, e.g. when the outcome is survival and mediation is via a process - in this case it can be shown that natural (in)direct effects are neither well-defined nor identifiable while separable effects are (Didelez, 2019). Further, separable effects lead to a new estimand for competing events settings: Well-known concepts are based on cause-specific incidence or on treating the competing event as censoring; these can be seen as total causal effect or as effect under an additional intervention eliminating the competing event. Instead, the separable direct effect on the event of interest is the effect of the treatment component that only activates causal paths avoiding the competing event (Stensrud et al., 2020).
Examples from the field of cancer research and drug development will be given. The presentation will focus on basic principles and concepts rather than technical details.
Thursday 23rd September, 4:30-5:30pm AEST (via Zoom).
References
- Andrews & Didelez (2021). Insights into the Cross-world Independence Assumption of Causal Mediation Analysis, Epidemiology: 32(2), 209-219.
- Didelez (2019). Defining causal mediation with a longitudinal mediator and a survival outcome. Lifetime Data Analysis 25, 593-610.
- Robins & Richardson (2011). Alternative graphical causal models and the identification of direct effects. In P. Shrout, K. Keyes, & K. Ornstein (Eds.), Causality and Psychopathology: Finding the Determinants of Disorders and Their Cures. Oxford University Press. 103–158.
- Stensrud, Young, Didelez, Robins & Hernán (2020) Separable effects for causal inference in the presence of competing events, JASA (online).