25 Sep 2014 10:00am
Conditional autoregressive models for geographically sparse outcomes
Seminar
Event Location
AustraliaDocuments

Links
Arul EarnestSpeakers
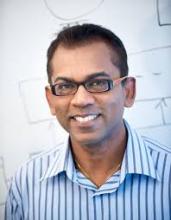
Arul Earnest
Monash University, School of Public Health and Preventive Medicine
Spatial epidemiology is the description and analysis of geographically indexed health data with respect to demographic, environmental, behavioral, socioeconomic, genetic, and infectious risk factors. Common familiar regression models are not sufficient to analyse such data, as they do not account for inherent spatial correlation in the outcomes and exposure variables. Sparse outcomes also add to the challenges in the analysis of such small-area datasets. Using a series of published papers, this talk will highlight one such Bayesian model, the Conditional Autoregressive (CAR) model, with practical applications to various health datasets from Australia and Singapore.